CISESS has announced four Seed Grant Program awards to help develop transformative research that requires a proof of concept to assist NOAA in recognizing and supporting new topics that eventually will be carried out in CISESS. The four projects selected are:
Building & Deploying Tools to Better Observe Lightning in Washington D.C. Region & Beyond – PI: Daile Zhang
This project will prepare a low-cost and innovative atmospheric electricity and lightning measurement tool and observation network to enhance the existing NOAA’s lightning networks including the Geostationary Lightning Mappers and Lightning Mapping Array, and provide complementary information of thunderstorm characteristics and lightning activity in the greater Washington D.C. area. It will also provide a great opportunity for students to have hands-on experience in fieldwork observation with the new science and technology and contribute to the NOAA advancement of instrumentation and technology growth. The new network will provide lightning videos at regular speed, and potentially high speed, 360-degree videos, as well as electric field waveforms. The data will be available at the CISESS Atmospheric Electricity Research and Training website that is still under development and testing. The design and the network deployment information will be uploaded to a Github repository.
Developing Low-Cost Microwave Radiometer for Student Training and Supporting JPSS Programs – PI: Hu Yang
This project will develop a low-cost microwave radiometer operating at Ka band (22 GHz). This instrument will be used for education and will include training materials to support effective use of JPSS microwave products such as TPW from ATMS. It can provide end-to-end demonstration and education for remote sensing user community, from principles of instrument observations to EDR product retrieval algorithms. It will aid users with different backgrounds to have a better understanding of the microwave remote sensing science in the JPSS program. It also will provide a platform for undergraduate and graduate students to be actively involved in different levels of the JPSS project, from instrument design, system integration and calibration, to geophysical parameters retrieval algorithm development. The instrument will be built in the Remote Sensing Lab at CISESS/UMD. Two different types of radiometers will be built under the project: a total power radiometer and a Dicke-type radiometer. The former follows the same operation principles as the JPSS ATMS instrument, and the latter is more suitable for a ground-based radiometer with high stability. All parts and components required to build the instruments will be purchased from domestic commercial market at low cost. Radiometer with the digital back-end will also be investigated if the funding support can be extended to the second year.
Real-Time NOAA Weather and Climate Product Analysis in Virtual Reality – PI: Guangyang Fang & Joseph Patton
The target of this project is to explore creative ways of using virtual reality (VR) to analyze and visualize 3-D NOAA weather and climate datasets in an immersive environment. The project consists of two main components: a preprocessing program and a visualization program. The preprocessing program ingests the NOAA weather and climate datasets, processes them to the format that can be ingested by the visualization software, and saves them to the filesystem. The visualization program allows users to select a specific dataset to be visualized inside of VR. The VR tools can supplement traditional forecasting and enhance forecasters’ ability to predict weather events. This project is based on the previous MeteoVis project, which aimed to explore the visualization of meteorological satellite and model data in an immersive VR environment. The figure shows the Advanced Layer Precipitation Water (ALPW) product Scene main control panel and 3D Earth with ALPW data visualized.

Emulating Microwave Measurements Using ABI Observations – PI: Veljko Petkovic
With a goal to maximize the benefits of current and future NOAA satellite observation capabilities, this project will study emulating passive microwave (PMW) observations using ABI radiances. The CISESS team, led by Dr. Veljko Petkovic, will use novel Machine Learning (ML) tools to bridge the gaps in spatio-temporal coverage of low Earth orbiting sensors. In close collaboration with Naval Postgraduate School (Monterey, CA), CISESS team has identified potential ABI observations that have the information content typically seen in passive microwave level-1 products. The pilot study will employ deep learning neural networks to emulate PMW observations within GOES-16 and -17 domain, targeting ABI spatial and temporal resolution under all weather conditions. The resulting product is expected to support a wide range of applications, including but not limited to nowcasting, data assimilation, satellite retrievals, and understanding of cloud microphysical processes. To ensure successful application of the emulated observations, the project will provide what is often seen as a major missing element of ML-based products –information on product’s uncertainty. Supported by CISESS postdoctoral associate, Dr. Malar Arulraj, and a visiting intern from University of California Irvine, the team will use modified architectures of deep learning systems to estimate errors of emulated PMW observations. An example of using ABI’s 2-, 10- and 16-channel radiances to emulate GMI 19H GHz channel brightness temperature is shown in the figure below (top panel). This demonstration is complemented by a showcase example of quantifying errors using the same ML model applied to a classification task where precipitation type is predicted using PMW observations (bottom panel). The initial results report high spatial coincidence between radiometer-observed and ABI-predicted (i.e., emulated) Tbs, and realistic estimates of ML-predicted uncertainties.
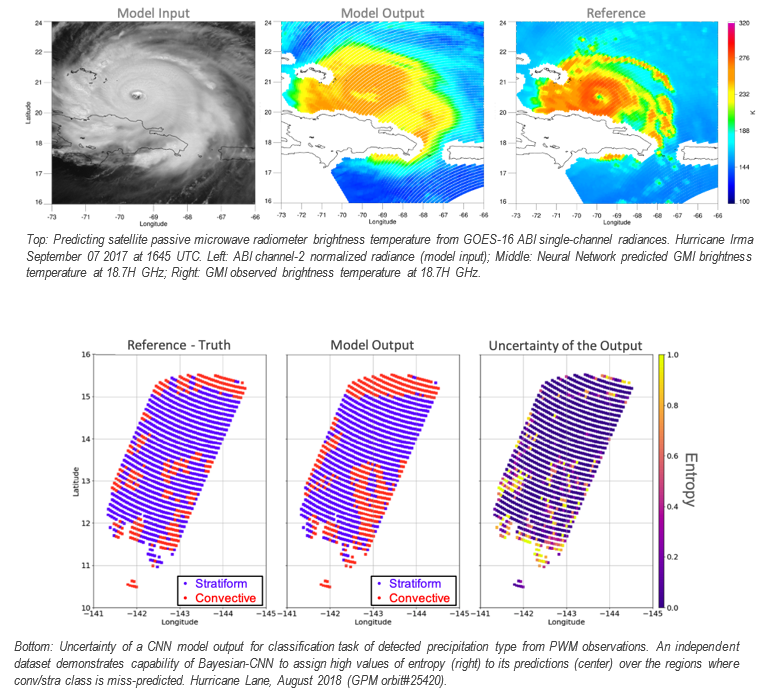
All projects begin on January 15.