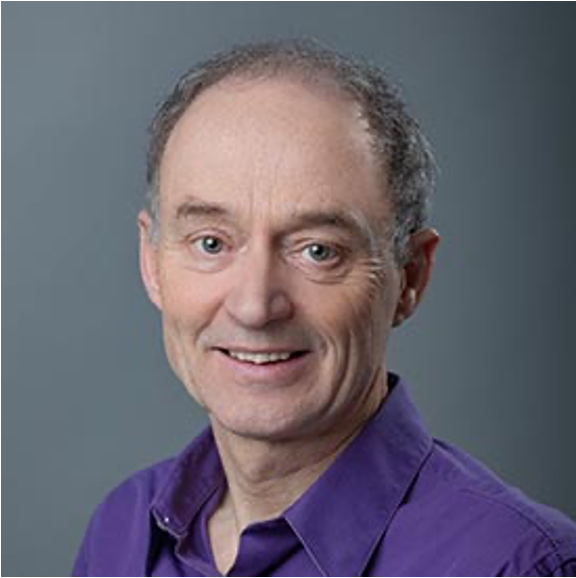
Improving Climate Models Using Corrective Machine Learning vs. Other Emulation Approaches
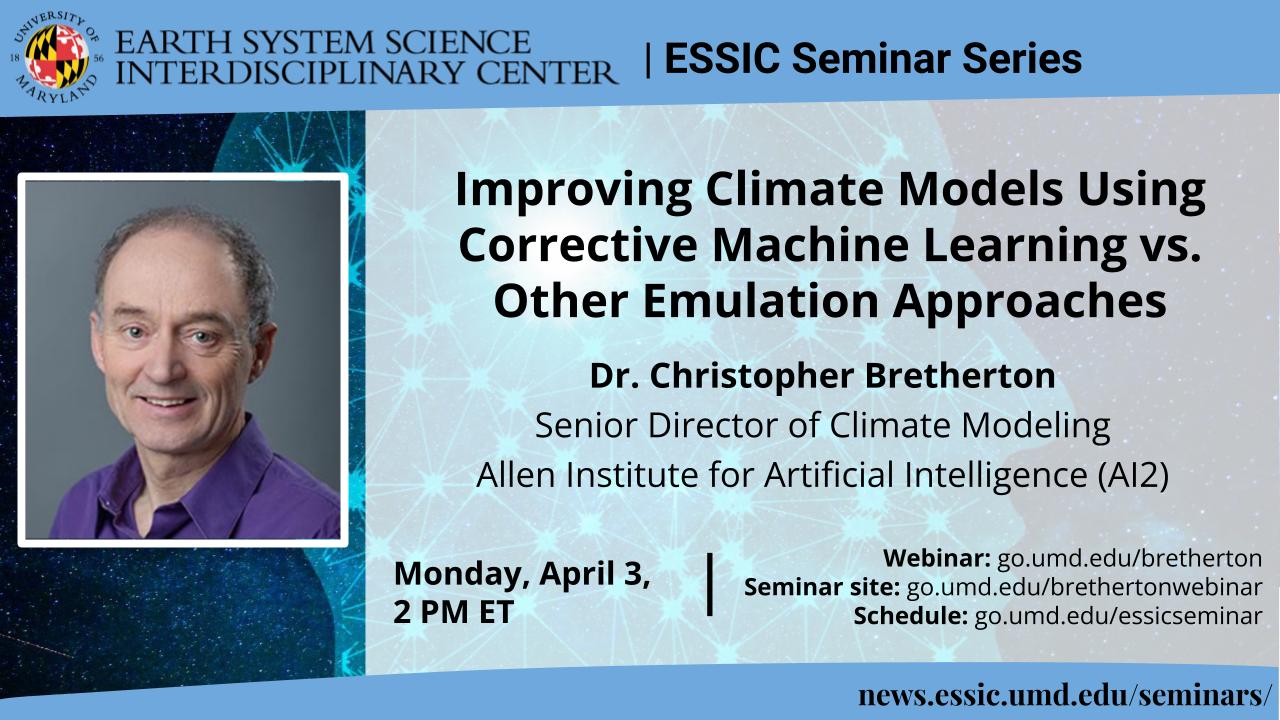
Dr. Christopher Bretherton
Senior Director of Climate Modeling
Allen Institute for Artificial Intelligence (AI2)
Monday April 3, 2023, 2 PM ET
Abstract:
AI2, with GFDL, has developed a corrective machine learning (ML) methodology to improve weather forecast skill and reduce climate biases in a computationally efficient coarse-grid climate model. The corrective ML is trained by nudging the 3D temperature, humidity and wind fields forecast by the coarse-grid model to a time-dependent global reference and learning the ‘nudging tendencies’ as a function of the column state of the model. The reference can be a reanalysis (for present-climate simulation) or a finer-grid version of the same model that may be more trustworthy across a range of climates. The ML is interpreted as a correction to the combined physics parameterizations of the coarse-grid model. We trained the ML on global 25 km simulations in multiple climates, and separately on a year-long 3 km simulation, and applied it in 200 km coarse-grid simulations. The ML reduced annual-mean land temperature and precipitation pattern biases by up to 50% and enhanced weather forecast skill. We compare strengths and weaknesses of this method vs. other strategies for emulating reference climate models, including another hybrid approach using reservoir computing, and full model emulation.
Biosketch:
Chris Bretherton directs a climate modeling group at AI2 in Seattle which uses machine learning trained on global storm-resolving model output and observational data to improve climate model simulations. He is an Emeritus Professor of the Atmospheric Science and Applied Mathematics Departments at the University of Washington, where for 35 years he studied cloud formation and turbulence and improved their simulation in atmospheric models. He is an American Meteorological Society Charney Award winner, IPCC author, AMS and AGU Fellow, and a member of the National Academy of Sciences.
Webinar:
Event site: https://go.umd.edu/bretherton
Zoom Webinar: https://go.umd.edu/brethertonwebinar
Zoom Meeting ID:
Zoom password: essic
US Toll: +13017158592
Global call-in numbers: https://umd.zoom.us/u/aMElEpvNu
For IT assistance:
Cazzy Medley: cazzy@umd.edu
Resources:
Seminar schedule & archive: https://go.umd.edu/essicseminar
Seminar Google calendar: https://go.umd.edu/essicseminarcalendar
Seminar recordings on Youtube: https://www.youtube.com/user/ESSICUMD