ESSIC/CISESS scientist Jifu Yin is the first author on a two-part series published in IEEE Transactions on Geoscience and Remote Sensing on refining the Advanced Microwave Scanning Radiometer 2 (AMSR2) soil moisture retrieval algorithm. The paper’s co-authors include ESSIC scientists Jicheng Liu, Huan Meng and Ralph Ferraro.
Soil moisture (SM) is an important variable for hydrological, meteorological, and agricultural studies and applications. NOAA-NESDIS developed the SM Operational Products System (SMOPS) to operationally provide an integrated satellite SM data product. AMSR2 SM retrieval is an important component of the currently operational SMOPS. This study is proposed to refine the AMSR2 data product using an optimal machine-learning model, and this first paper of the two-part series is to intercompare the six commonly used machine-learning models: multiple linear regression (MLR), regression tree (RRT), random forest (RFT), gradient boosting (GBR), extreme gradient boosting (XGB), and artificial neural network (ANN). The researchers found that the XGB method is the most successful with respect to the reference data SM Active Passive (SMAP) and the in situ observations from the U.S. Department of Agriculture Soil Climate Analysis Network (SCAN).
In the second paper, the researchers train the XGB model using AMSR2 brightness temperature measurements in dual polarizations, ancillary maps, and the vegetation index datasets and in turn used to predict the daily global AMSR2 SM retrievals from 2012 to 2021. They found that the refined AMSR2 SM retrievals (AMSRr) show an overwhelming advantage in data accuracy over the currently operational AMSR2 (AMSRc) product. Compared to the AMSRc, the developed AMSRr presents a significant improvement on data availability.
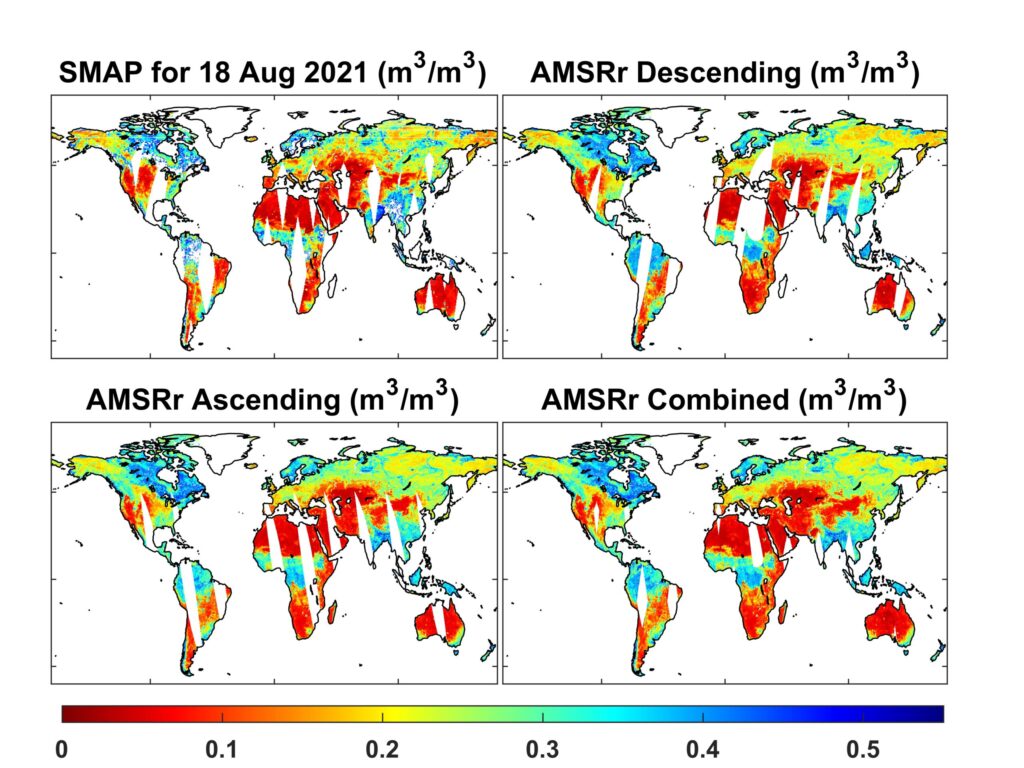
Based on this study, higher quality AMSRr SM data products will be operationally produced in the NOAA and will eventually benefit the NOAA SMOPS blended SM product and its users.
Yin is currently an Associate Research Scientist with University of Maryland’s Earth System Science Interdisciplinary Center. His research interests include data assimilation, drought monitoring, microwave satellite soil moisture retrievals and land surface model.
Liu is a Visiting Scientist with the NOAA-NESDIS. His current research interests include developing soil moisture retrieval algorithms using data from microwave satellite sensors.
Meng is a physical scientist with NOAA/NESDIS Center for Satellite Applications and Research, Satellite Climate Studies Branch, and a Visiting Research Scientist at ESSIC. She received a MS in Physical Oceanography from Florida State University in 1993, and a PhD in Hydrology from Colorado State University in 2004. She has been working in the field of satellite remote sensing since 1999. Her current research focuses on snowfall retrieval using passive microwave measurements from polar-orbiting satellites.
Ferraro is the Associate Director of ESSIC and former Chief of the NOAA/NESDIS Satellite Climate Studies Branch. Ferraro’s current research focuses on the use of environmental satellite remote sensing for both weather and climate studies with an emphasis on precipitation and other hydrological cycle products.
To access the papers, click here: