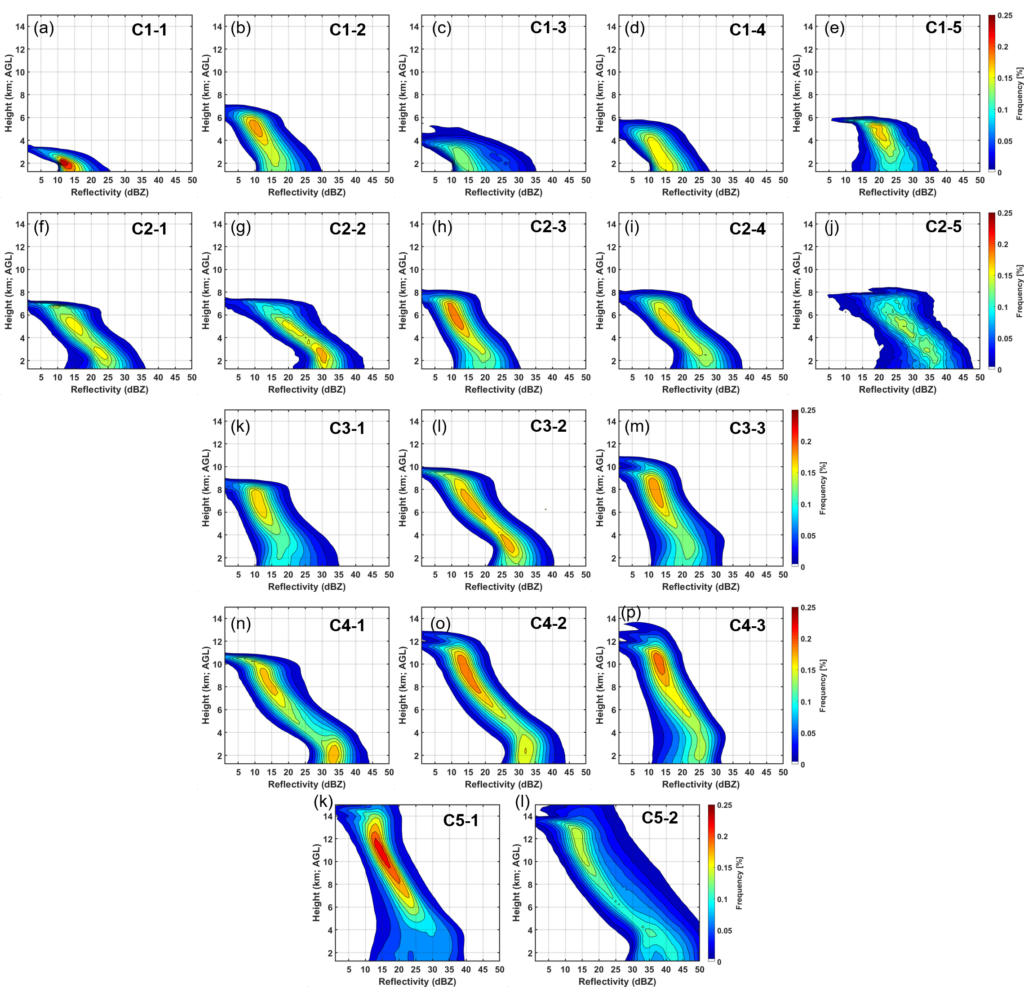
ESSIC/CISESS scientists Malarvizhi Arulraj, Veljko Petkovic, Ralph R. Ferraro, and Huan Meng have a new study out in Journal of Hydrometeorology titled, “Precipitation Vertical Structure Characterization: A Feature-Based Approach”.
The three-dimensional (3D) structure of precipitation systems is highly dependent on hydrometeor formation processes and microphysics. This study aims to characterize distinct vertical profiles of precipitation regimes by relying on the availability of a high-quality, spatially dense radar network and its capability to observe the 3D structure of the storms. The researchers used six months of the 3D spatially dense precipitation data from the Multi-Radar Multi-Sensor (MRMS) network as the training dataset for a deep-learning-based neural network. This deep-learning system was then used to identify different patterns or regimes in the vertical structure of precipitation systems based on clustering methods.
The framework found 18 regimes within the data that consists of more physical information than the usual classifications of convective vs. stratiform. These regimes are now being studied to determine what we can learn from them about precipitation systems.
To access the article, click here: “Precipitation Vertical Structure Characterization: A Feature-Based Approach”.