The Snowfall Rate (SFR) team at STAR and ESSIC/CISESS, including Huan Meng, Yongzhen Fan, and Jun Dong, has made some notable progress recently that significantly enhances the product and its accessibility to users.
The team developed machine learning (ML) snowfall detection (SD) algorithms, and improved snowfall rate estimation through ML-enhanced initialization and bias correction for the NOAA-20 and S-NPP satellites. The new SD models enable SFR retrieval in extremely cold conditions including the polar regions. The previous SD models required 2 m temperature to be above -15 °C (5 °F). In addition, they extended the current land-only SFR product to over ocean including sea ice and coast. This makes the JPSS SFR a global product, which meets the JPSS requirements. Effort is ongoing to develop ML models for the POES and Metop satellites.
Finally, the team has developed a new product website (http://sfr.umd.edu). The page is designed to be user friendly and has a range of functionalities to facilitate usability. The SFR product from 5 satellites is produced at CISESS in near real-time using direct broadcast data from University of Wisconsin. Product images are overlaid on Google Maps on the new website. Besides SFR, the website also features an experimental radar-satellite merged snowfall rate product, mSFR. The figure below shows NOAA-20 SFR capturing the intense lake effect snow on November 18, 2022 (left) and the corresponding gauge-corrected Multi-Radar Multi-Sensor (MRMS) precipitation analysis (right).
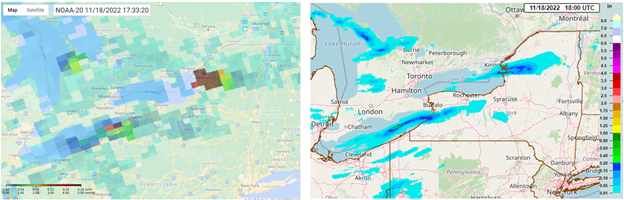
Meng is a physical scientist with NOAA/NESDIS Center for Satellite Applications and Research, Satellite Climate Studies Branch, and a Visiting Research Scientist at ESSIC. She received a MS in Physical Oceanography from Florida State University in 1993, and a PhD in Hydrology from Colorado State University in 2004. She has been working in the field of satellite remote sensing since 1999. Her current research focuses on snowfall retrieval using passive microwave measurements from polar-orbiting satellites.
Fan received the B.S. degrees in electronic science from Xi’an University of Science and Technology, Xi’an, Shaanxi, China in 2004 and the Ph.D. degree in Physics from Stevens Institute of Technology, Hoboken, NJ, USA in 2016. He is currently an Associate Research Scientist with the Earth System Science Interdisciplinary Center (ESSIC) and the Cooperative Institute for Satellite Earth System Studies (CISESS)-Maryland, University of Maryland (UMD), College Park, MD, USA. His research interests include radiative transfer theory, machine learning, satellite remote sensing of ocean color, aerosols and snowfall.
Dong is a faculty specialist at Earth System Science Interdisciplinary Center (ESSIC) and Cooperative Institute for Satellite Earth System Studies (CISESS)-Marylandof, University of Maryland. He received his Ph.D degree in Geophysical Fluid Dynamics from Florida State University in 2012. He has over fifteen years of experience in Methodology, Physical Oceanography and Remote Sensing. At ESSIC/CISESS, his work focuses on microwave satellite snowfall rate (SFR) retrieval algorithm development, implementation and real-time SFR product maintenance.