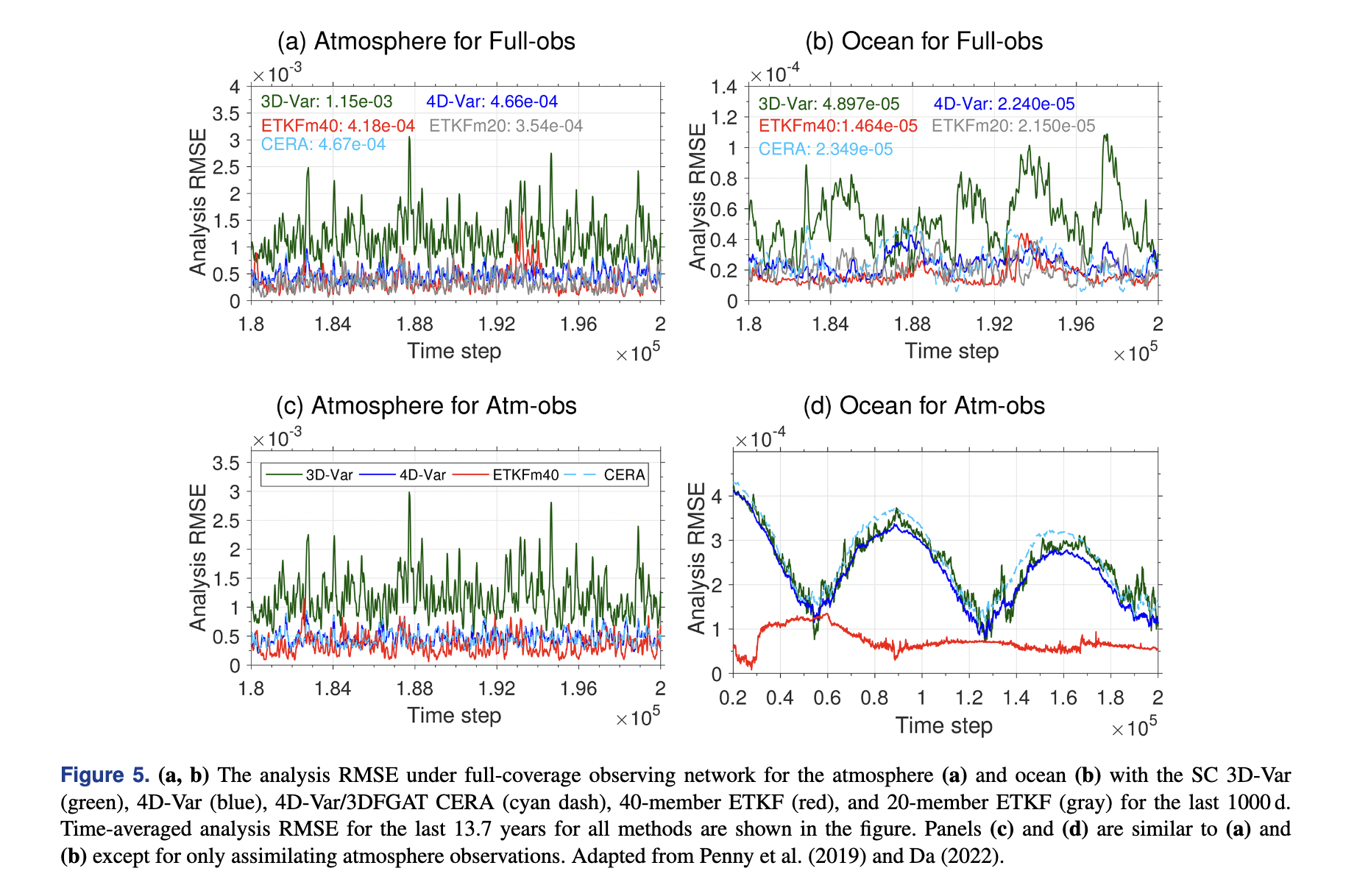
A team of international researchers led by ESSIC Scientist Distinguished University Professor Eugenia Kalnay and including Dr. Cheng Da recently reviewed their latest research progress on coupled data assimilation in a new paper published in Nonlinear Processes in Geophysics titled, “Review article: Towards strongly coupled ensemble data assimilation with additional improvements from machine learning”.
Recently, the weather and climate modeling community have been tremendously interested in coupled data assimilation (CDA), with many operational centers planning to use CDAs to make seamless weather-climate prediction models. The team’s 2016 & 2018 studies have shown that a strongly coupled data assimilation (SCDA) approach, which creates coupled analyses by assimilating the same set of the all-domain observations into different Earth system components, outperforms a weakly coupled data assimilation (WCDA) approach in terms of the analysis accuracy and observation departures.
In this paper, Kalnay, Da, and their co-authors explore the benefits of SCDA over other CDA strategies using a wide range of coupled models with increasing complexities, ranging from the simple coupled Lorenz model to the state-of-the-art operational coupled model CFSv2.
With the Lorenz model, the team proved that SC EnKF and 4D-Var could constrain the fast and slow modes of the coupled model simultaneously. Experiments with a coupled quasi-geostrophic (QG) model show that SCDA produces more accurate analyses than other CDA strategies for both variational and ensemble methods. In addition, SC ETKF shows persistent, smaller ocean analysis errors than WC ETKF, a phenomenon not observed for 3D-Var. Comparison of SCDA approaches under a full observing network shows that EnKF, 4D-Var and ECMWF’s CERA-approach reach similar analysis accuracy. Real-observation experiments with the CFSv2-LETKF demonstrated that assimilating additional lower atmosphere observations into the ocean through SCDA improves the analysis of the atmosphere and upper ocean but degrades observation fits in the deep ocean, probably due to the unreliable error correlation estimated by a small ensemble.
The team then developed the correlation-cutoff method that improves the performance of SCDA for a small ensemble. For applications to the operational models, a neural network approach is proposed to systematically acquire the observation localization needed by this method.
While the study focuses on coupled atmosphere-ocean analyses, the SCDA approach is promising for other coupled components, such as coupled land-atmosphere data assimilation. CDA can also be applied to coupled Earth-human systems, where Earth system components are coupled with human system components using bidirectional feedback. Dynamical models of the human system are not yet broadly developed, leading to uncertainties when making projections using coupled models. CDA will be a crucial method to quantify and constrain these uncertainties.
Kalnay is a Distinguished University Professor with ESSIC, Atmospheric and Oceanic Sciences, Civil and Environmental Engineering, and Mechanical Engineering. Current research interests of Kalnay are in numerical weather prediction, data assimilation, predictability and ensemble forecasting, coupled ocean-atmosphere modeling, climate change, and sustainability. She has also published several highly cited papers on the need to model the bidirectional coupling that exists between the Human and the Earth Systems.
Da is a postdoc associate at UMD ESSIC and Global Modeling and Assimilation Office at NASA Goddard Space Flight Center, working on coupled data assimilation. His research interests are in coupled and satellite data assimilation. Before joining ESSIC in 2022, he obtained his Ph.D. in atmospheric and oceanic science from University of Maryland, focusing on non-Gaussian assimilation of NASA GPM-derived surface precipitation for improved hurricane predictions. Da was also a recipient of the NASA Earth and Space Science Fellowship since 2018.
To access the paper, click here: “Review article: Towards strongly coupled ensemble data assimilation with additional improvements from machine learning”.